Now is a great time to build a pure-play data (DaaS) business
Even with ZoomInfo’s success, there are surprisingly few DaaS start-ups
There are hundreds of amazing companies that sell software and tools to data scientists and machine learning teams. In fact, many of the best companies in the last 15 years have been exactly that.
But outside of SafeGraph (where I work), there are almost no companies that specialize in selling data to data scientists.
Why?
Partially it is because it is MUCH easier to get to $10 million ARR by selling applications (traditional SaaS). Partially it is just tradition coupled with stagnation. Partially it is because venture capital firms have been wary of funding data companies. And, most convincingly, being a data-only business is less exciting to most entrepreneurs because data is a supporting role (see the last section on data and humility).
But selling data to data scientists is starting to be a big business.
Selling data (DaaS or Data-as-a-Service) historically has not been a great business. Outside of Zoominfo and a few others, there have been almost no pure-play data unicorns built in the last 20 years.
Check out the DaaS Bible -- the ins and outs of running a data-as-a-service business (DaaS accounting, winner-take-most dynamics, and more)
That’s because very few companies had the ability to make use of raw data in the past. 10 years ago, only the most advanced engineering teams were able to make use of external data. But that’s changing. An order of magnitude more companies buy data today than did five years ago. That’s because a good engineer with a tool like Snowflake can be as productive as a great engineer was 5-10 years ago.
This is happening across industries.
One example is hedge funds. Not that long ago, just ten funds were buying significant alternative data. Today it is still under 100. But there are 500-700 funds that are currently making the investment to ingest large amounts of data.

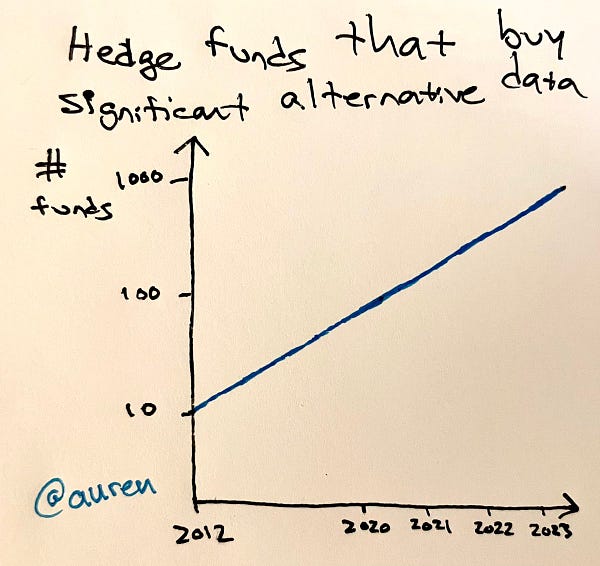
The last 15 years has been about how companies get insights from their own data -- and many of the great companies played into that trend -- Tableau, Palantir, Databricks, etc. But the most advanced organizations are now reaching an asymptote where there’s only marginal value they can get from mining just their own data.
Companies that are far along the curve of getting insights from their own data need external data if they want to continue to get value from their data science expertise. Because even mighty Walmart only knows about 0.1% of the world from their internal data alone.
These data scientists have lots of people selling them applications. Some of the most valuable companies are ones that sell tools to these data scientists. But outside of SafeGraph, they have been severely neglected in getting high-quality data. Almost no one is creating data for these data scientists.
But the market is there now.
Three of the companies that became SafeGraph customers last month (at $324k ARR, $225k ARR, and $500k ARR) were not even buying data a year ago. So the market for buying high-quality data is growing rapidly … and it is still super new.
So while data businesses have historically been sub-scale businesses (most companies that had proprietary data become applications), now is the time to build a world-class pure-play data company. We’re just at the inflection point.
Check out Why Data Standards Matter - I co-wrote this with Will Lansing - CEO of FICO. Gives an overview of how data standards make data more valuable.
What kind of data do data scientists need?
First, and most importantly, they need data that is actually true. A lot of data one can buy is 50-60% true. That kind of data can be very valuable for marketing -- where 60% true is much better than throwing darts.
But 60% precision does not pass the quality bar for data science and machine learning teams … because when you are building models, errors compound quickly.
For instance, let’s say you are building an image recognition system to tell the difference between cats and dogs. Having a truth set of labelled photos of cats and dogs would be very helpful. It would be a much bigger challenge if the data was only 60% correct -- because, as mentioned, errors can compound quickly.
Ideally you want the truth set to be true. Of course, it will never be 100% true. But you want to get it to as close to 100% as possible.
Data and humility
As eluded to above, the biggest reason that there are not more data companies is that DaaS is a true supporting role. Data companies support the true innovators. Data companies are like archivists and librarians.
I tell everyone at SafeGraph that we are selling high-quality butter to pastry chefs. The end consumer of the pastry might not even know there is butter in the pastry (and they certainly won’t know it is SafeGraph branded butter). But the chef knows how important we are.
Of course, the most important player in making the pastry is the chef. She is the one combining the dozens of ingredients together to make a delicious pastry. And therein lies the humility one needs to have when at a data company: we are just one of many important ingredients that goes into a creation.
Summation: now is the time to build a pure-play data business with data scientists as your customers.
Here are three Napkin Graphs from the last week (and enjoy more in my Twitter feed):
And two tweets:
Great read Auren. One problem that I've noticed about this space is that it's very difficult to curate differentiated data. As a DAAS business, how do you identify what is differentiated data, and how do you curate that to interested parties? More importantly, how do clients authenically discover and trust your data? As someone who provides data services, I wonder how many people come across my data and say "I don't know if I can trust this."
I'd love your opinion!
We see so many data conversations and data conferences around data pools and data dashboards. However, very few organizations invest in 'metadata strategy' which makes their data useful and meaningful for internal or external use. By 2025, I guess "metadata strategist" should take over the what we have now as 'Data scientist'.